♪苫小牧オケ練習 2007.11.18(日)12:00-16:45@アイビープラザホール
曲 第九 1→3→2→4楽章 → ヘンデル ラルゴ
・この日は苫小牧第九の、合唱も入った総練習。合唱とは初合わせになります。普段のアイビープラザの音楽室ではなく、人数が多いのでホールでの練習でした。
・前半はオケのみで1から3楽章の練習。ほぼ全ての楽器が揃う。
・3楽章:鳥肌たつようなホルンソロ、Kさんさすがです。第九全楽章を弾くのは3度目ですが、特定パートの抜き出し練習なんかで「まだこんなメロディーが隠れてたのか!」という発見がチラホラ。
・2楽章:某氏の指摘により、遅めだった中間部のテンポを早めに変更。途端に音楽がサラサラと流れ出す。全然弾きやすい。いわゆる『普通のテンポ』という先入観があるのはあまりよろしくないですが、やはり『弾きやすいテンポ』というものは存在していて、「遅くすれば、合う」というのは必ずしも成り立たないことを実感しました。
・合唱の声だし準備のため、オケは30分ほど休憩。この日はあちこちから手伝いの人が来ていて、ロビーは各地の奏者で賑わい、『アマオケサロン』さながら。室蘭のオケでは見なくなってしまった、室蘭在住の方がたくさん混じっていました。Vn、Vc、Ob、Pic、Trb…などなど。室蘭ではなく苫小牧でご一緒するとは、なんか変な感じ。
・4楽章:合唱はずいぶんたくさんいました。ステージ上に山台をおいても、後ろの人は指揮が見えてるんだかいないんだか。全員で強奏すると、さすがに迫力があります。まずは歌の入るあたりから始めて、最後まで通し。後で部分的にチェック。合唱は1時間ほどで解放され、残りの時間は4名のソリストとの合わせをしました。ソリストはSopとBarは昨年の定演で共演した方で、Tenは欠席のため代役です。
それにしてもこの曲、合唱の出番少ないよなぁ。。。
・練習後は急いで札幌市民オケの練習へ向かう。
・演奏会のチラシには前回の苫小牧での第九の集合写真が使われています[写真]。日付は平成5年10月9日。オケをよ~く見てみると、室蘭から手伝いに行っていたO師匠をはじめとするなつかしい顔ぶれやら、ハネケンさんなんかが写っています。なんてったって14年前、皆さん小さい写真でも判別できるくらいに若い! 私がまだバイオリンをはじめて数ヶ月のころです。今回の写真がまた次回の第九に使われることになるのでしょうか。
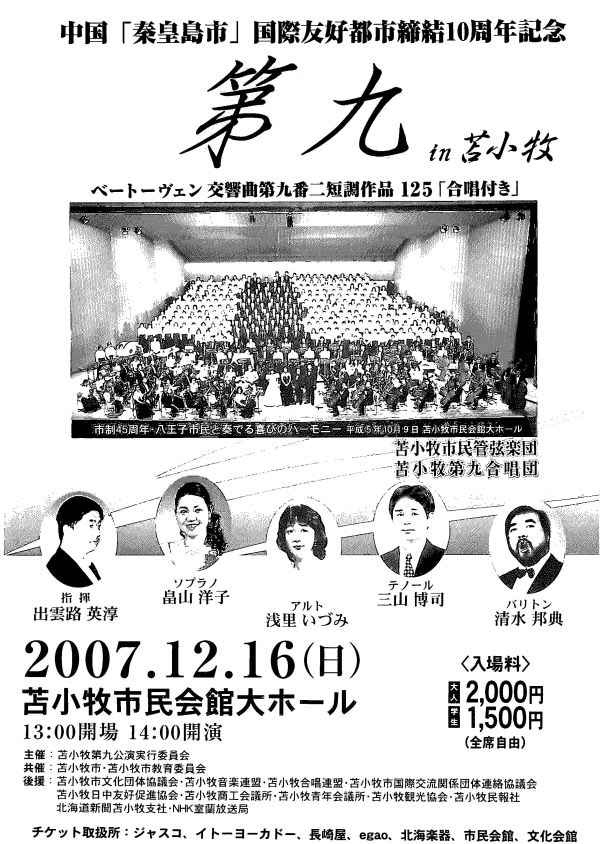
♪本番 中国「秦皇島市」国際友好都市締結10周年記念 第九in苫小牧 2007.12.16(日)14:00開演@苫小牧市民会館
曲 第九 1→3→2→4楽章 → ヘンデル ラルゴ
・この日は苫小牧第九の、合唱も入った総練習。合唱とは初合わせになります。普段のアイビープラザの音楽室ではなく、人数が多いのでホールでの練習でした。
・前半はオケのみで1から3楽章の練習。ほぼ全ての楽器が揃う。

・2楽章:某氏の指摘により、遅めだった中間部のテンポを早めに変更。途端に音楽がサラサラと流れ出す。全然弾きやすい。いわゆる『普通のテンポ』という先入観があるのはあまりよろしくないですが、やはり『弾きやすいテンポ』というものは存在していて、「遅くすれば、合う」というのは必ずしも成り立たないことを実感しました。
・合唱の声だし準備のため、オケは30分ほど休憩。この日はあちこちから手伝いの人が来ていて、ロビーは各地の奏者で賑わい、『アマオケサロン』さながら。室蘭のオケでは見なくなってしまった、室蘭在住の方がたくさん混じっていました。Vn、Vc、Ob、Pic、Trb…などなど。室蘭ではなく苫小牧でご一緒するとは、なんか変な感じ。
・4楽章:合唱はずいぶんたくさんいました。ステージ上に山台をおいても、後ろの人は指揮が見えてるんだかいないんだか。全員で強奏すると、さすがに迫力があります。まずは歌の入るあたりから始めて、最後まで通し。後で部分的にチェック。合唱は1時間ほどで解放され、残りの時間は4名のソリストとの合わせをしました。ソリストはSopとBarは昨年の定演で共演した方で、Tenは欠席のため代役です。
それにしてもこの曲、合唱の出番少ないよなぁ。。。
・練習後は急いで札幌市民オケの練習へ向かう。
・演奏会のチラシには前回の苫小牧での第九の集合写真が使われています[写真]。日付は平成5年10月9日。オケをよ~く見てみると、室蘭から手伝いに行っていたO師匠をはじめとするなつかしい顔ぶれやら、ハネケンさんなんかが写っています。なんてったって14年前、皆さん小さい写真でも判別できるくらいに若い! 私がまだバイオリンをはじめて数ヶ月のころです。今回の写真がまた次回の第九に使われることになるのでしょうか。
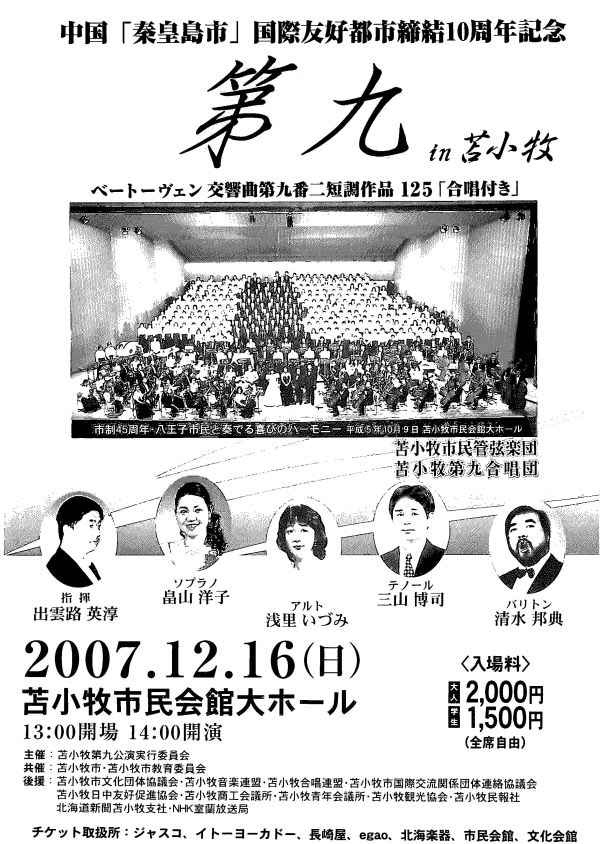
♪本番 中国「秦皇島市」国際友好都市締結10周年記念 第九in苫小牧 2007.12.16(日)14:00開演@苫小牧市民会館